The Acoustic Emotion Gaussians Model for Emotion-based Music Annotation and Retrieval
Proceedings of ACM Multimedia 2012, 89-98
Ju-Chiang Wang, Yi-Hsuan Yang, Hsin-Min Wang, and Shyh-Kang Jeng
- Institute of Information Science, Academia Sinica
One of the most exciting but challenging endeavors in music research is to develop a computational model that comprehends the affective content of music signals and organizes a music collection according to emotion. In this paper, we propose a novel acoustic emotion Gaussians (AEG) model that defines a proper generative process of emotion perception in music. As a generative model, AEG permits easy and straightforward interpretations of the model learning processes. To bridge the acoustic feature space and music emotion space, a set of latent feature classes , which are learned from data, is introduced to perform the endto-end semantic mappings between the two spaces. Based on the space of latent feature classes, the AEG model is applicable to automatic music emotion annotation, emotion-based music retrieval, and emotion-based music video composition. Our results show that the AEG model outperforms the state-of-the-art methods in automatic music emotion annotation. This work won the first prize of the 2012 ACM Multimedia Grand Challenge.
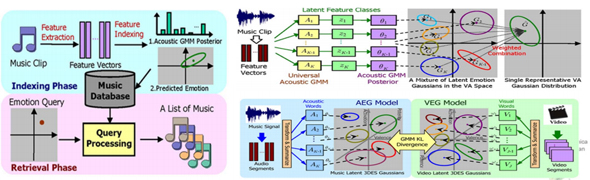
Online Optimization with Gradual Variations
Journal of Machine Learning Research - Proceedings Track 23 (COLT), 2012, 6.1-6.20
Chao-Kai Chiang, Tianbao Yang, Chia-Jung Lee, Mehrdad Mahdavi, Chi-Jen Lu, Rong Jin, and Shenghuo Zhu
- Institute of Information Science, Academia Sinica
Many situations in daily life require ones to make repeated decisions in an unknown and changing environment, including examples such as trading stocks, forecasting weather, commuting to work, playing games, etc. This motivates the study of the so-called online optimization problem in which a player must iteratively choose an action and receive a loss for a number of rounds. We consider a natural restriction of this problem in which the loss functions have a small deviation, to mode l environments which evolve gradually. For this, we provide online algorithms which achieve smaller regret bounds than previous ones.
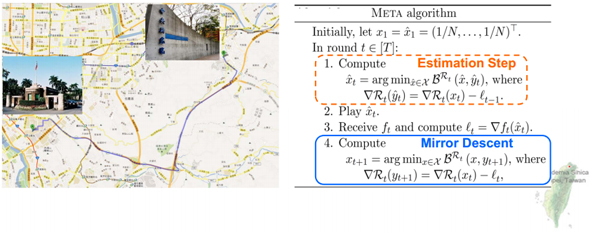
Image Feature Extraction in Encrypted Domain with Privacy-preserving SIFT
IEEE Transactions on Image Processing 2012, 21(11), 4593-4607
Chao-Yung Hsu, Chun-Shien Lu, and Soo-Chang Pei
- Institute of Information Science, Academia Sinica
Privacy has received considerable attention but is still largely ignored in the multimedia community. Consider a cloud computing scenario where the server is resource-abundant, and is capable of finishing the designated tasks. It is envisioned that secure media applications with privacy preservation will be treated seriously. In view of the fact that scale-invariant feature transform (SIFT) has been widely adopt ed in various fields, this paper is the first to target the importance of privacy-preserving SIFT (PPSIFT) and to address the problem of secure SIFT feature extraction and representation in the encrypted domain. As all of the operations in SIFT must be moved to the encrypted domain, we propose a privacy-preserving realization of the SIFT method based on hom omorphic encryption. We show through the security analysis based on the discrete logarithm problem and RSA that PPSIFT is secure against ciphertext only attack and known plaintext attack.
